AI ML Solutions, Automation Services, Web & Mobile App Development Solutions, Software Development Services
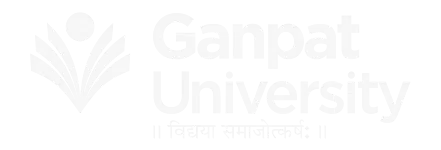
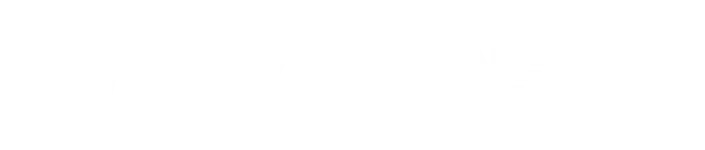
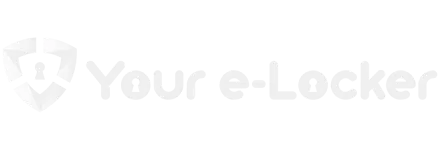
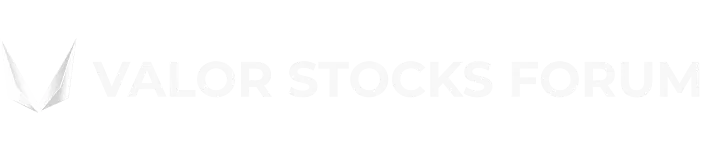
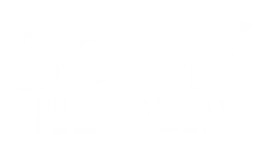
AI ML services
Driving The Next Generation Of
Smart AI Solutions
Our full-spectrum AI ML services empower your business to stay ahead in tech landscape.
Computer Vision for AR/VR Experiences
At Webelight Solutions, we harness AI-driven computer vision technology to enhance visual search & create immersive AR VR experiences that engage users effectively.
Revolutionary AI-Powered Super Apps
We develop AI-powered super apps that combine multiple functionalities into a single platform, driving AI transformation to boost customer satisfaction & engagement.
NLP Solutions for Sentiment Analysis
Our AI-enabled Natural Language Processing solutions provide insights into customer sentiment, helping businesses make informed decisions for future-ready strategies.
Process Automation with RPA & ML
We leverage next-gen AI, robotic process automation, and machine learning to automate repetitive tasks, streamline operations, optimize workflows & boost productivity.
AI Chatbots with Emotion Detection
Our AI chatbots feature advanced emotion detection, enabling AI strategy & personalized interactions that improve customer service by understanding user emotions.
AI-Driven Infrastructure Optimization
We specialize in AI-driven infrastructure optimization to ensure efficient, scalable systems that maximize performance while significantly reducing operational costs.
Our Services
Artificial Intelligence Solutions
Harness advanced AI technology to automate tasks, analyze data, and create intelligent AI systems to enhance customer experiences, optimize operations, and drive business innovation.

Machine Learning Solutions
Leverage AI-driven data algorithms to predict trends, optimize processes, and deliver AI-ready experiences, enabling smarter decision-making and improving efficiency across various industries.

Custom Software Development
Tailored AI software solutions designed to ensure seamless integration, enhanced functionality, and scalable growth, optimizing your organization and products with custom AI solutions.

Mobile App Development
Delivering high-performance AI-powered mobile apps tailored to ensure engaging user experiences, and compatibility across devices, driving growth in the AI mobile-first world.

DevOps Solutions
Streamline AI-enabled software development process through cloud technologies, continuous integration, and smart AI strategy to automate workflows leading to reduced time to market.

Advanced Automation
Employ cutting-edge AI technology to automate repetitive tasks, reduce errors to help businesses simplify complex processes, optimize workflows, and lead to AI transformation.

Synergizing Industry Needs
Bespoke AI Solutions
Designed For Diverse Industries
Our dedicated team of trained AI specialists ensures that businesses worldwide across every industry receive tailored AI solutions.
Why Webelight Solutions?
Making Impossible Achievable
with Cutting-Edge AI Capabilities
We blend AI expertise with your business insight by leveraging advanced AI solutions & a decade of experience in AI/ML strategies.
What you can expect from us
Access our expert team with 10+ years of AI-driven startup and product success.
Tailored AI-powered product development plans aligned with your business goals.
Cutting-edge Next-Gen AI solutions and digital expertise to drive your project forward.
AI-powered scalable solutions that grow with your business, adapting to new needs.
Continuous support & clear communication to keep you engaged at every step.
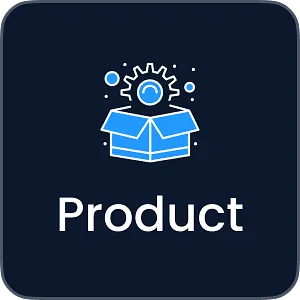
What we expect from you
A well-defined idea of your business goals, objectives, and desired outcomes.
Active collaboration throughout the project, with AI-driven insights and feedback.
Regular updates & transparent communication to ensure alignment address concerns.
Timely access to resources, including information, materials, and decision-makers.
Confidence in our AI expertise and flexibility to adapt to strategic recommendations.
Our Company Overview
Global
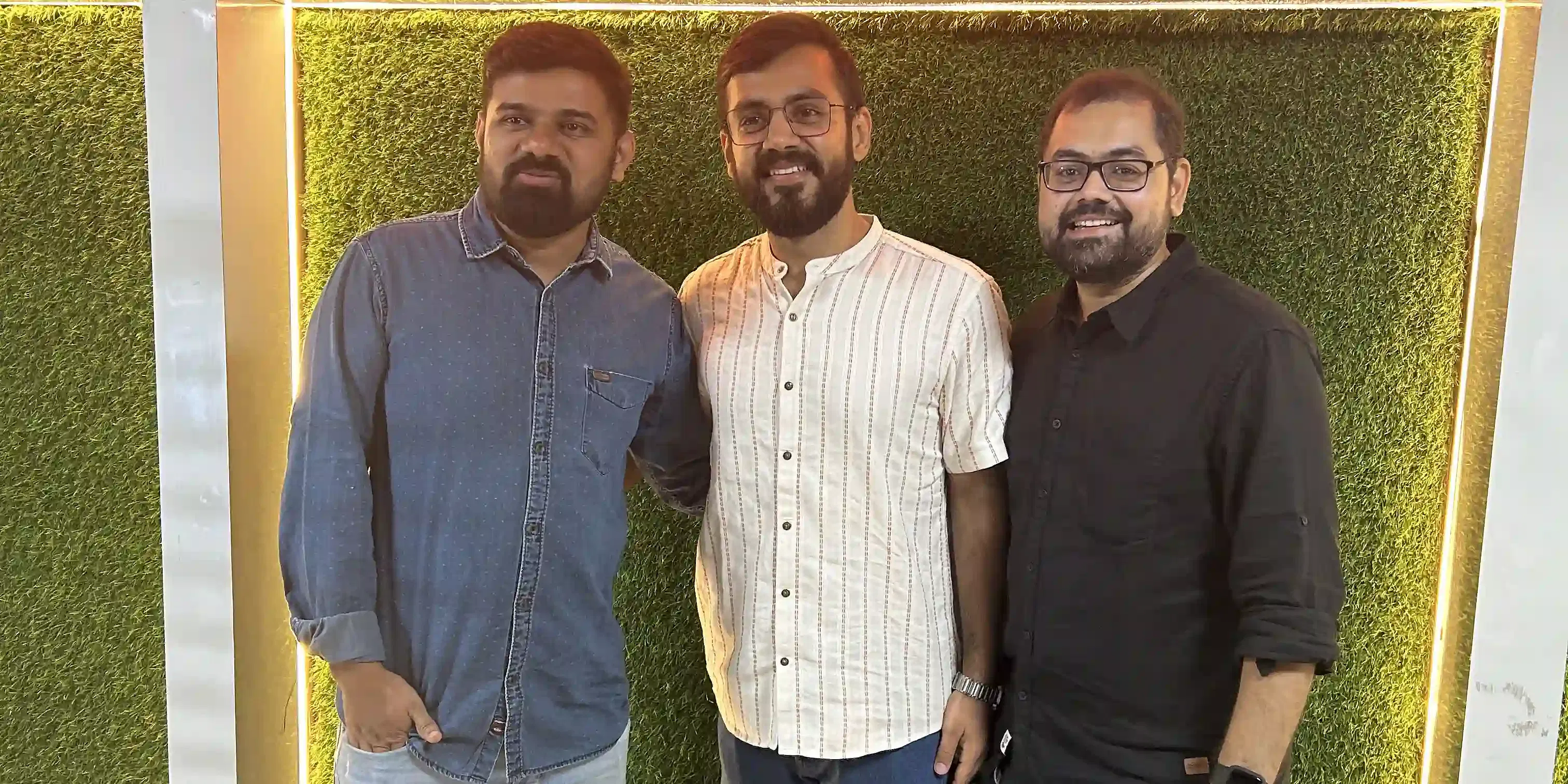
10+
Years of Experience
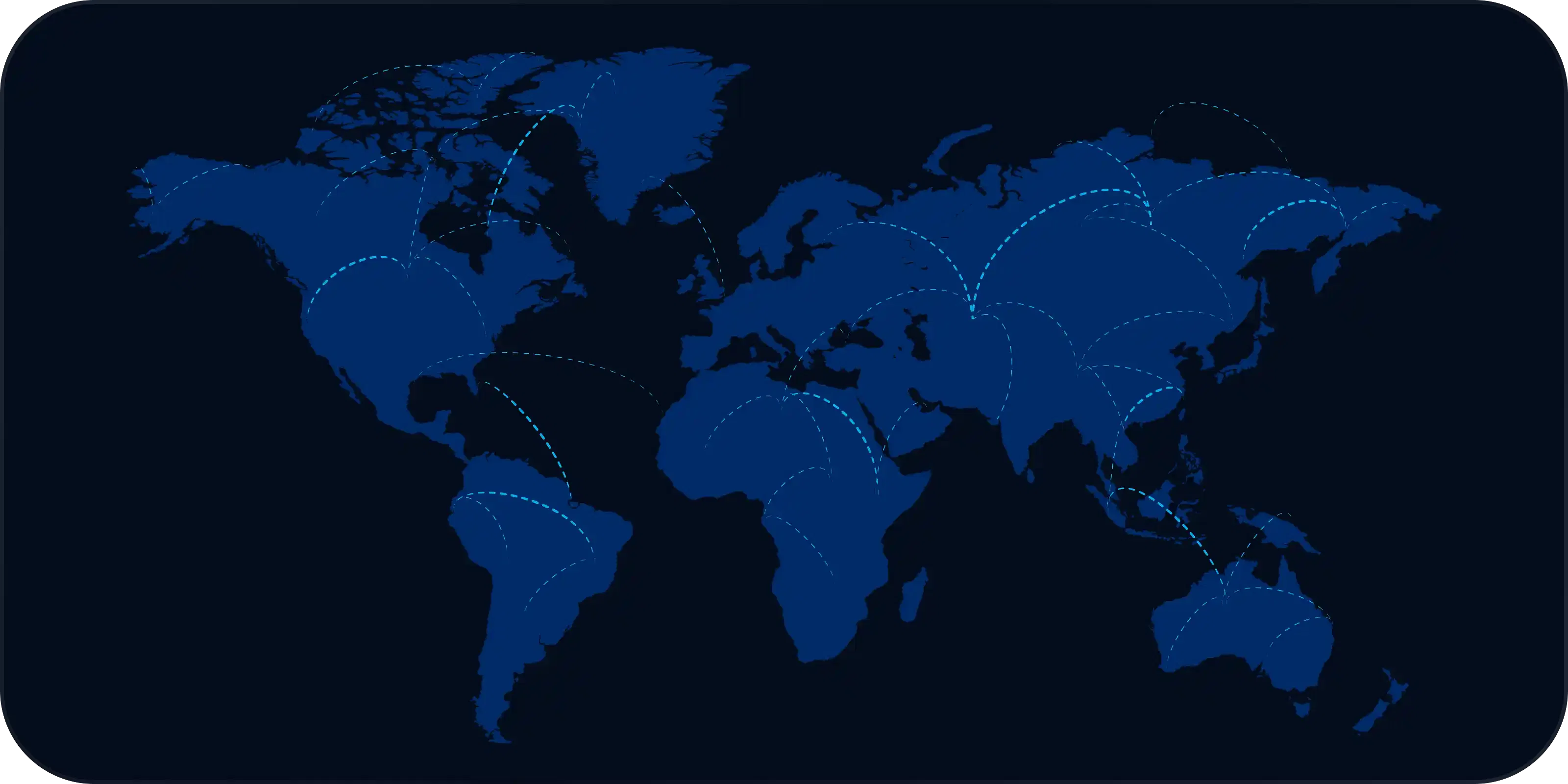
25+
Countries Served
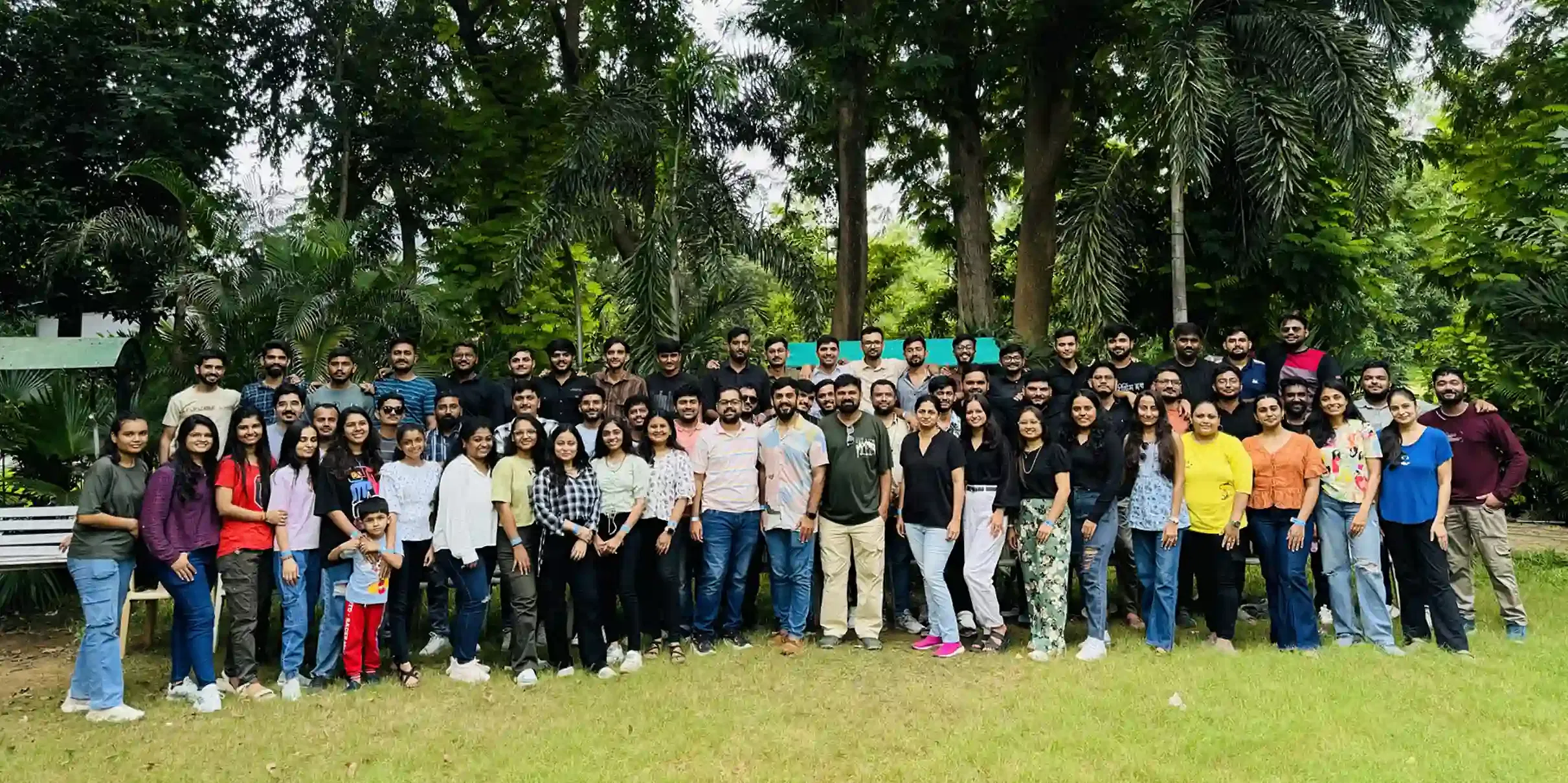
110+
AI Specialists
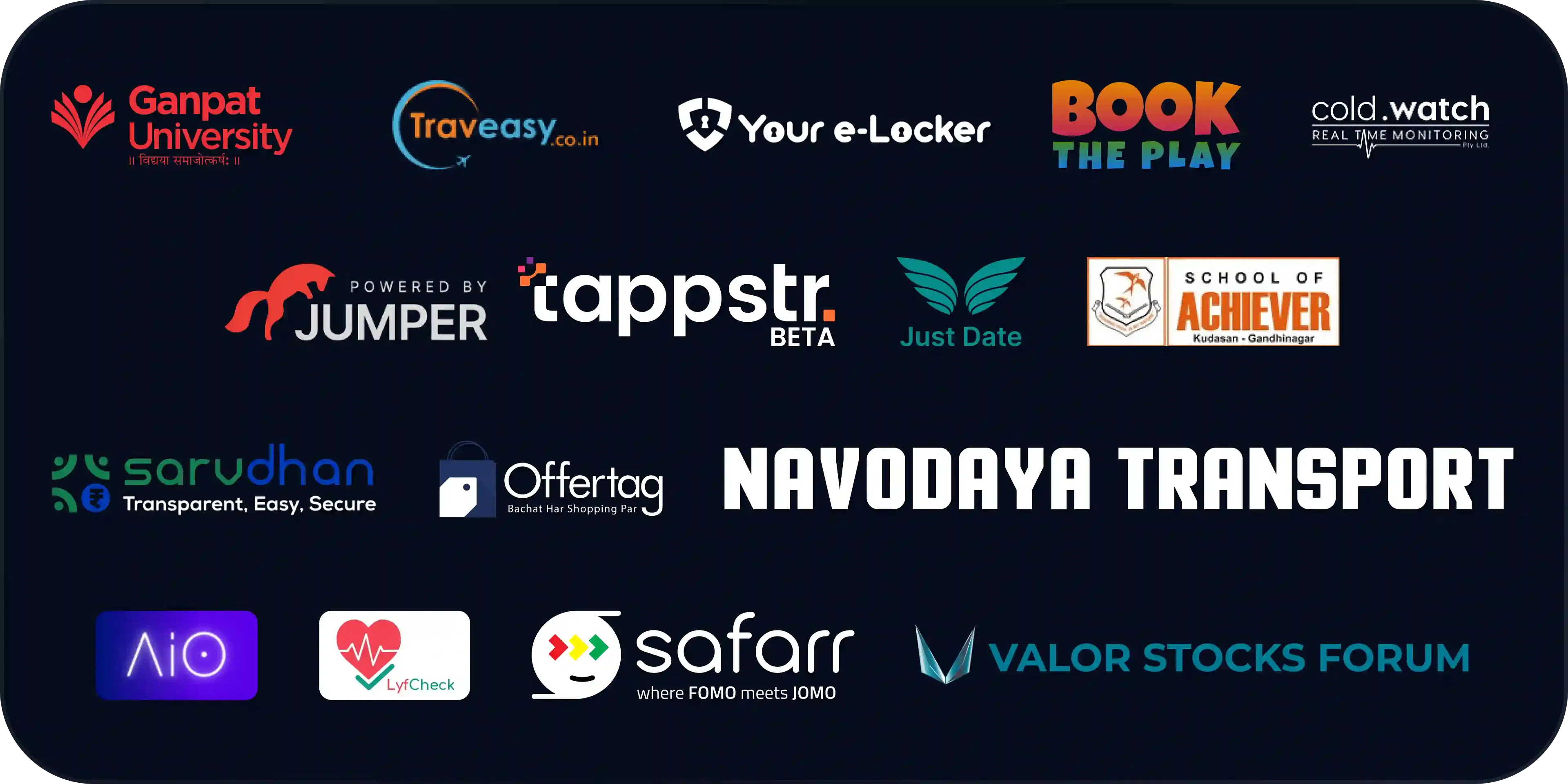
500+
Products Delivered
The AI Innovation Engine
Our Latest
AI Technology Stack
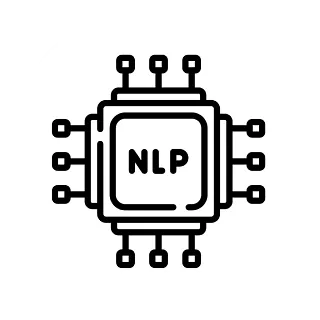
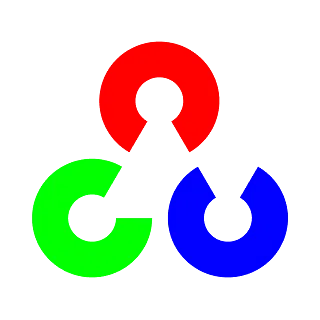
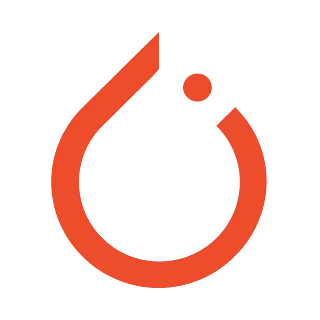
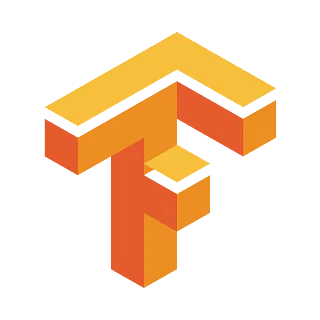
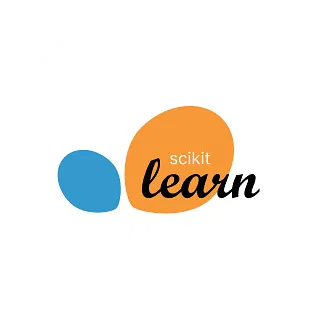

Testimonials
Empowering our clients' success with
cutting-edge AI Solutions
Highly technical, dedicated, and truly goes above and beyond to meet client needs.
We have been working with Jayesh, Yash, and Miraj since the inception of Webelight, and I must say, this trio is exceptional. Their commitment to delivering the best solutions is evident in every project they handle. The team is highly technical, dedicated, and truly goes above and beyond to meet client needs. We wish Webelight continued success and growth in the years to come!

Zeel Patel
Head IT, School of Achiever
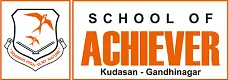
They responded fast, communicated well, and were committed to delivering high-quality work
The scope of work included UI/UX design, front-end and back-end development, API integration, cross-platform compatibility (iOS and Android), performance optimization, bug fixing, testing, and final deployment. Regular updates were provided throughout the development process to ensure alignment with project goals.They responded fast, communicated well, and were committed to delivering high-quality work, which made the experience great.

Ilzira AS
Strategy & Development Director, AdamSea
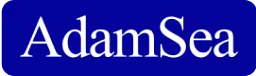
Loading blog posts...
FAQs
Common Questions
We've compiled a list of frequently asked questions with clear and concise answers.